LLMs Transforming Pharma and Medical Sciences
- AlphaSquare Labs Content Desk
- Jan 2
- 4 min read
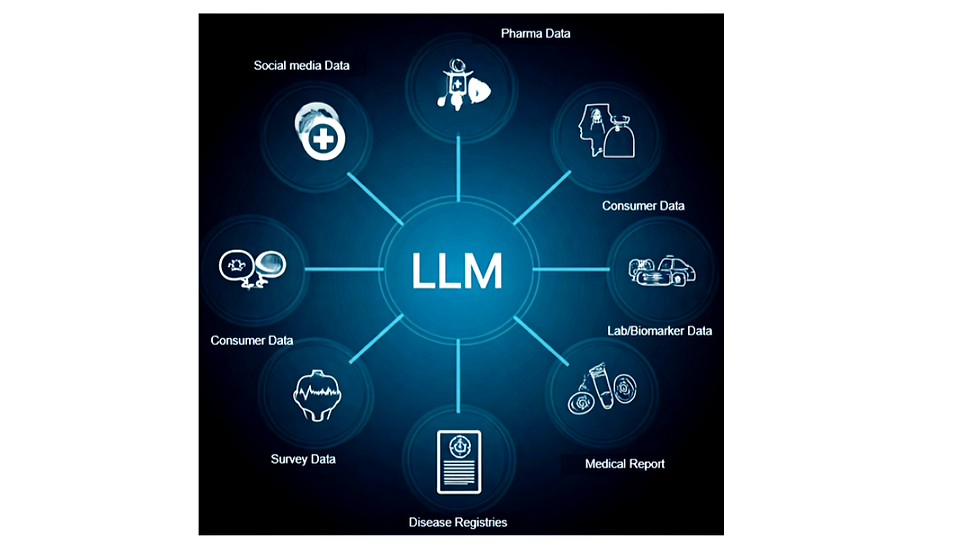
Large Language Models (LLMs) are transforming the pharmaceutical and medical sciences industries by automating the analysis of vast biomedical data, accelerating drug discovery, and enhancing clinical decision-making. These advanced AI models interpret complex medical literature, offering invaluable insights to researchers and healthcare professionals. In this article, we explore leading LLMs like BioGPT and PubMedGPT, highlighting their technical foundations, applications, and their impact on the medical field.
Overview of Prominent LLMs in Pharma and Medical Sciences
Several LLMs are specifically designed for applications in the medical and pharmaceutical sectors. These models are trained on vast biomedical datasets and tailored for tasks such as drug discovery, clinical decision support, and scientific literature analysis. Here's a brief overview of some key models:
BioGPT (Microsoft): Focuses on processing biomedical text, excelling in drug discovery, clinical decision support, and literature summarization. Its strength lies in its specialized training on biomedical literature, giving it deep domain expertise.
PubMedGPT (Meta): Trained on PubMed data, this model excels in summarizing medical research, conducting literature reviews, analyzing clinical trials, and generating hypotheses.
MedPaLM (Google): Aimed at answering medical questions accurately, MedPaLM is useful in clinical decision-making and patient care support, handling complex medical queries effectively.
ClinicalBERT: Designed for clinical data analysis, ClinicalBERT helps predict patient outcomes and analyze electronic health records (EHRs) with its fine-tuned approach.
MedGPT: Specializes in generating medical content, such as reports and clinical documentation, simplifying the often complex process of medical writing.
Galactica (Meta): Focuses on synthesizing scientific knowledge by analyzing literature and testing hypotheses, providing advanced summarization capabilities.
SciBERT: Tailored for scientific text processing, SciBERT is used in text classification and entity recognition, particularly in research contexts.
GatorTron (NVIDIA): Optimized for healthcare-specific text, GatorTron excels in extracting data from clinical notes, enhancing patient management.
In-Depth Analysis of Leading LLMs
1. BioGPT (Microsoft)
BioGPT is designed to efficiently process and interpret biomedical data, playing a critical role in drug discovery, clinical decision support, and literature summarization.
Technical Architecture:
Model Type: BioGPT utilizes a transformer-based architecture that has been fine-tuned specifically for biomedical content.
Components: -
Attention Mechanism: Enhances context comprehension by focusing on the most important words within a sentence.
Pre-Training and Fine-Tuning: Initially trained on general datasets, BioGPT is further fine-tuned on biomedical texts to improve its domain-specific performance.
Specialized Tokenization: Handles complex medical terms and abbreviations accurately, which is critical in the medical field.
Key Applications:
Drug Discovery: BioGPT identifies links between chemical compounds and biological targets, which accelerates hypothesis generation and reduces R&D costs.
Clinical Decision Support: By comparing patient data with a vast repository of medical knowledge, BioGPT can suggest clinical interventions, enhancing diagnostics and enabling personalized treatments.
Literature Summarization: It efficiently summarizes lengthy research articles, saving time for researchers and providing quick access to critical insights.
Technical Strengths and Challenges:
Strengths: BioGPT is highly adept at managing specialized biomedical terminology and complex sentence structures. Its contextual sensitivity allows it to recognize subtle nuances in scientific literature.
Challenges: The model is susceptible to data biases, particularly as it is predominantly trained on Western biomedical literature. Additionally, the high computational demands for training and deployment can pose scalability issues for smaller institutions.
2. PubMedGPT (Meta)
PubMedGPT is a specialized LLM that synthesizes large volumes of medical literature, primarily focusing on data from PubMed. It plays a significant role in automating literature reviews, analyzing clinical trials, and generating research hypotheses.
Technical Architecture:
Model Type: PubMedGPT uses a transformer model optimized for medical content.
Components:-
Domain-Specific Fine-Tuning: Trained specifically on PubMed data, giving it deep expertise in medical research.
Advanced Tokenization: Efficiently manages medical terms, abbreviations, and drug names.
Sequence Modeling: Generates coherent summaries of scientific articles, making complex research data accessible.
Key Applications:
Automated Literature Review: PubMedGPT scans and synthesizes findings from vast numbers of research papers, streamlining systematic reviews and keeping researchers updated.
Clinical Trial Analysis: The model analyzes and summarizes clinical trial data for specific conditions, supporting evidence-based planning in clinical settings.
Hypothesis Generation: It identifies research gaps, suggesting new study directions and fueling scientific discovery.
Technical Strengths and Challenges:
Strengths: PubMedGPT delivers high accuracy in biomedical contexts and links its responses to existing literature, ensuring the credibility of its outputs.
Challenges: The model's performance is limited by the quality and scope of the data from PubMed, and its outputs often require expert review to avoid inaccuracies.
Conclusion
LLMs like BioGPT and PubMedGPT are revolutionizing the pharmaceutical and medical sciences by providing advanced AI-driven insights into drug discovery, clinical decision-making, and medical research. Their specialized architectures enable precise handling of biomedical literature, setting new standards for efficiency and innovation in healthcare. Despite challenges such as data biases and high computational costs, these models are paving the way for a new era in medical research and patient care. Future articles will explore other LLMs that continue to drive advancements in this critical field.
Comments